is the most important staple food crop which feeds over half of the world population.
It also has emerged as a reference plant for monocotyledonous species including many cereals due to its desirable attributes as a model crop:
relatively compact genome size, well annotated genome, abundant functional genomics data, and well-established methods for genetic transformation.
In addition, genome-scale libraries of targeted loss-of-function or gain-of-function mutants have enabled functional analysis of rice genes.
is still challenging in rice.
Despite the effective reverse-genetics resources and transformation methods, complicated many-to-many relationships between genes and complex traits of crop species makes it troublesome.
The unbiased genome-wide reverse-genetic analysis, hence, would be ineffective.
Thus, gene prioritization methods and algorithms to enrich true trait-associated genes for the functional tests are in high demand.
has been proven to be successful in genetics for many species including rice.
Previously, we have demonstrated that a genome-scale gene network for Oryza sativa, RiceNet (www.functionalnet.org/ricenet)
can effectively prioritize the candidate genes modulating innate immunity in rice,
and have validated three novel regulators of biotic stress response
(Lee et al. 2011).
is an improved network prioritization web server for Oryza sativa.
(1) The co-functional network for prediction has been substantially improved by using improved machine learning algorithms as well as by incorporating a large amount of new genomics data. We compared RiceNet v2 with the previous network using validation set of gene pairs from AgriGO annotations which is independent from network training data. We observed significantly improved quality of the network and the prediction power by RiceNet v2 (p-value = 1.11e-6 by Wilcoxon signed rank test; see figures below).
(2) It makes predictions for both major subspecies of rice, japonica and indica,
while the previous server could only make predictions for japonica.
(3) Users can now use Arabidopsis genes as guide genes for prioritization. This is a useful option because many trait-associated genes are evolutionarily conserved between rice and Arabidopsis. We can take advantage of the extensive number of Arabidopsis genes-to-trait annotations to infer rice trait-associated genes.
(4) It uses a new algorithm of network prioritization, context-associated hub, which uses differentially expressed genes (DEGs) as input data. This would be useful in prediction of rice genes associated with stress response.
(5) All component networks integrated into the RiceNet v2 are downloadable. This enables users to construct alternative network models by using different data integration algorithms.
(1) The co-functional network for prediction has been substantially improved by using improved machine learning algorithms as well as by incorporating a large amount of new genomics data. We compared RiceNet v2 with the previous network using validation set of gene pairs from AgriGO annotations which is independent from network training data. We observed significantly improved quality of the network and the prediction power by RiceNet v2 (p-value = 1.11e-6 by Wilcoxon signed rank test; see figures below).
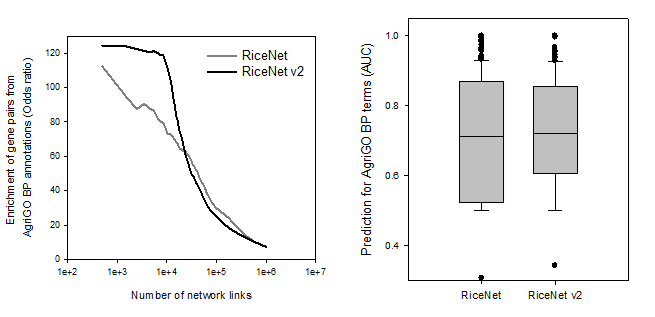
(3) Users can now use Arabidopsis genes as guide genes for prioritization. This is a useful option because many trait-associated genes are evolutionarily conserved between rice and Arabidopsis. We can take advantage of the extensive number of Arabidopsis genes-to-trait annotations to infer rice trait-associated genes.
(4) It uses a new algorithm of network prioritization, context-associated hub, which uses differentially expressed genes (DEGs) as input data. This would be useful in prediction of rice genes associated with stress response.
(5) All component networks integrated into the RiceNet v2 are downloadable. This enables users to construct alternative network models by using different data integration algorithms.
starts by submitting input data, a set of guide genes.
Gene prioritization based on network direct neighborhood option ranks rice genes from the most closely associated to the guide genes by direct neighborhood.
Based on the ranks of the guide genes, the server measures prediction power of the RiceNet v2 for the guide genes using ROC analysis.
Gene prioritization based on context-associated hubs option takes input DEGs which represent a biological context such as abiotic or biotic stress response, and then prioritize hub genes associated to the context.
Search result pages output candidate genes for both subspecies of rice, japonica and indica.
RiceNet v2: an improved network prioritization server for rice genes, Nucl. Acids Res. doi:10.1093/nar/gkv253, First published online: March 26, 2015 link

2014.12.29 RiceNet v2 website launched
This work was supported by the National Research Foundation of Korea grant (2010-0017649, 2012M3A9B4028641, 2012M3A9C7050151).
This work was also supported in part by the Joint BioEnergy Institute, the Office of Science, Office of Biological and Environmental Research, U.S. Department of Energy under Contract No. DE-AC02-05CH11231, and the Department of Energy Systems Biology Knowledgebase (KBase).